Contact
Positions
Publications
QRM
Software
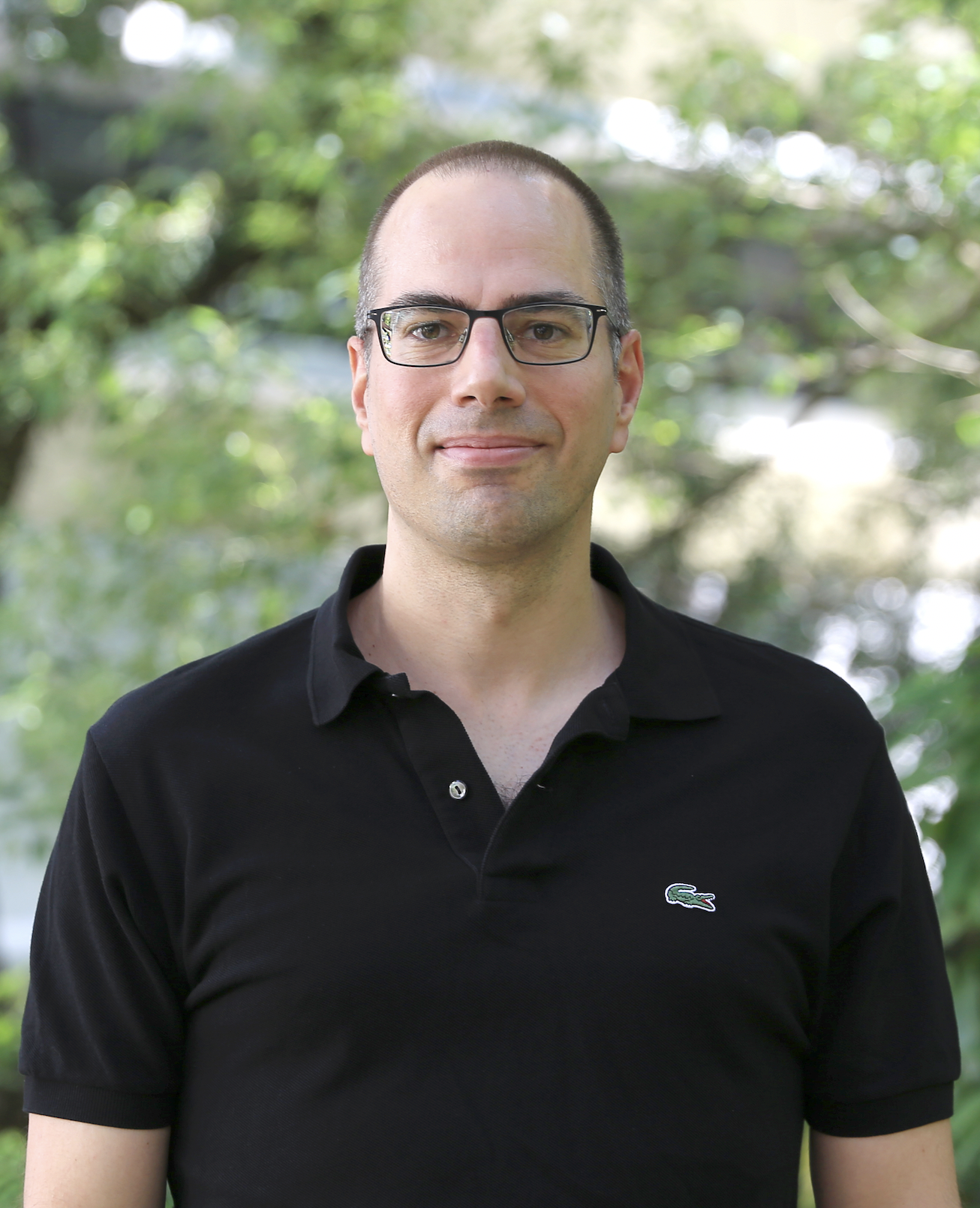
Marius Hofert, Dr. rer. nat.
Associate Professor of Statistics
Department of Statistics and Actuarial Science
Faculty of Science
The University of Hong Kong
Office: Room 228, Run Run Shaw Building
Email: mhofert at hku dot hk
URL: Departmental website
Google Maps: How to find me
Google Scholar:
My profile
My research interests are dependence modeling, computational statistics, data science and quantitative risk management. I am an Associate Editor of:
I am also the Director of HKU's Master of Data Science (MDASC) program and Academic Adviser in Risk Management.
For any inquiries concerning MDASC, please contact our supporting staff Clara Lian or Jacey Yeung directly
(see
here).
I have a
PhD position available. I am looking for a
mathematically strong, independent student interested in probability or statistics.
Knowledge of Git and LaTeX is required. If you are interested,
please send me your CV, transcripts (Bachelor's, Master's; including
a GPA for each degree) and some of your written work (for example
your Master's thesis).
Articles
[73]
Dong, G. Y., Hintz, E., Hofert, M. and Lemieux, C. (2024),
Randomized Quasi-Monte Carlo Methods on Triangles: Extensible Lattices and Sequences,
Methodology and Computing in Applied Probability, 26(15),
doi.org/10.1007/s11009-024-10084-z.
[72]
Liu, Y., Hofert, M. (2024), Policy optimization by looking ahead for model-based offline reinforcement learning, 41st IEEE Conference on Robotics and Automation (ICRA 2024), accepted.
[71]
Liu, Y., Hofert, M. (2024), Implicit and explicit policy constraints for offline reinforcement learning, Proceedings of Machine Learning Research, accepted.
[70]
Herrmann, K., Hofert, M., Sadr, N. (2023),
Index-mixed copulas, submitted (see also
here).
[69]
Koike, T., Hofert, M. (2023), Comparison of correlation-based measures of
concordance in terms of asymptotic variance, Journal of Multivariate Analysis, accepted.
[68]
Górecki, J., Hofert, M. (2023),
Composite pseudo-likelihood estimation for pair-tractable copulas such as Archimedean, Archimax and related hierarchical extensions,
Journal of Statistical Computation and Simulation, 93(13), 2321–2355,
doi.org/10.1080/00949655.2023.2180511.
[67]
[66]
[65]
Hofert, M. (2023),
The philosophy of copula modeling: A conversation with ChatGPT,
Journal of Data Science, 21(4), 619–637,
doi.org/10.6339/23-JDS1114.
[64]
Hofert, M., Prasad, A., Zhu, M. (2023),
Dependence model assessment and selection with DecoupleNets,
Journal of Computational and Graphical Statistics, 32(4), 1272–1286,
doi.org/10.1080/10618600.2022.2157835.
[63]
Hofert, M., Prasad, A., Zhu, M. (2022),
RafterNet: Probabilistic predictions in multi-response regression,
The American Statistician, 77(4), 406–416,
doi:10.1080/00031305.2022.2141857.
[62]
Koike, T., Hofert, M. (2022),
Matrix compatibility and correlation mixture representation of generalized Gini's gamma,
The Canadian Journal of Statistics, 51(4), 1111–1125,
doi.org/10.1002/cjs.11748.
[61]
Koike, T., Kato, S., Hofert, M. (2023),
Measuring non-exchangeable tail dependence using tail copulas,
ASTIN Bulletin, 53(2), 466–487,
doi.org/10.1017/asb.2023.4.
[60]
Coblenz, M., Grothe, O., Herrmann, K., Hofert, M. (2022),
Smooth bootstrapping of copula functionals,
Electronic Journal of Statistics, 16(1), 2550–2606,
doi.org/10.1214/22-EJS2007.
[59]
Hintz, E., Hofert, M. and Lemieux, C. (2022),
Multivariate Normal Variance Mixtures in R: The R Package nvmix,
Journal of Statistical Software, 102(2), 1–31,
doi.org/10.18637/jss.v102.i02.
[58]
Hintz, E., Hofert, M., Lemieux, C., Taniguchi, Y. (2022),
Single-Index Importance Sampling with Stratification,
Methodology and Computing in Applied Probability,
doi.org/10.1007/s11009-022-09970-1.
[57]
Hintz, E., Hofert, M., Lemieux, C. (2022),
Computational challenges of t and related copulas,
Journal of Data Science, 20(1), 95–110,
doi.org/10.6339/22-JDS1034.
[56]
Hofert, M., Prasad, A., Zhu, M. (2022),
Multivariate time-series modeling with generative neural networks,
Econometrics and Statistics, 23, 147–164,
doi:10.1016/j.ecosta.2021.10.011.
[55]
[54]
Hintz, E., Hofert, M., Lemieux, C. (2021),
Normal variance mixtures: Distribution, density and parameter estimation,
Computational Statistics & Data Analysis, 157,
doi:10.1016/j.csda.2021.107175.
[53]
[52]
Górecki, J., Hofert, M., Holeňa, M. (2020),
Hierarchical Archimedean Copulas for MATLAB: The HACopula Toolbox,
Journal of Statistical Software, 93, 10,
doi:10.18637/jss.v093.i10.
[51]
[50]
Hofert, M., Prasad, A., Zhu, M. (2021),
Quasi-random sampling for multivariate distributions via generative neural networks,
Journal of Computational and Graphical Statistics, 30(3), 647–670,
doi:10.1080/10618600.2020.1868302.
[49]
Górecki, J., Hofert, M., Okhrin, O. (2020),
Outer power transformations of hiearchical Archimedean copulas: Construction, sampling and estimation,
Computational Statistics & Data Analysis, 155, 107109,
doi:10.1016/j.csda.2020.107109.
[48]
Hofert, M., Oldford, R. W. (2019),
Zigzag expanded navigation plots in R: The R package zenplots,
Journal of Statistical Software, 95(4),
doi:10.18637/jss.v095.i04.
[47]
Hintz, E., Hofert, M., Lemieux, C. (2020),
Grouped Normal Variance Mixtures,
Risks, 8(4), 103,
doi:10.3390/risks8040103.
[46]
Hofert, M. (2020),
Random number generators produce collisions: Why, how many and more,
The American Statistician, 75(4), 394–402,
doi:10.1080/00031305.2020.1782261.
[45]
Hofert, M. (2020),
Implementing the Rearrangement Algorithm: An Example from Computational Risk Management,
Risks, 8(2), 47,
doi:10.3390/risks8020047.
[44]
Koike, T., Hofert, M. (2020),
Markov Chain Monte Carlo Methods for Estimating Systemic Risk Allocations,
Risks, 8(1), 6,
doi:10.3390/risks8010006.
[43]
Herrmann, K., Hofert, M., Mailhot, M. (2019),
Multivariate geometric tail- and range-value-at-risk,
ASTIN Bulletin, 50(1), 265–292,
doi:10.1017/asb.2019.31.
[42]
Hofert, M., Koike, T. (2019),
Compatibility and attainability of matrices of correlation-based measures of concordance,
ASTIN Bulletin, 49(3), 885–918,
doi:10.1017/10.1017/asb.2019.13.
[41]
Hofert, M., Oldford, R. W., Prasad, A., Zhu, M. (2019),
A framework for measuring association of random vectors via collapsed random variables,
Journal of Multivariate Analysis, 172, 5–27,
doi:10.1016/j.jmva.2019.02.012.
[40]
Hofert, M., Oldford, R. W. (2018),
Visualizing Dependence in High-dimensional Data: An Application to S&P 500 Constituent Data,
Econometrics and Statistics, 8, 161–183,
doi:10.1016/j.ecosta.2017.03.007.
[39]
Hofert, M., Huser, R., Prasad, A. (2018),
Hierarchical Archimax copulas,
Journal of Multivariate
Analysis, 167, 195–211,
doi:10.1016/j.jmva.2018.05.001.
[38]
Herrmann, K., Hofert, M., Mailhot, M. (2018),
Multivariate geometric expectiles,
Scandinavian Actuarial Journal, 2018(7), 629–659,
doi:10.1080/03461238.2018.1426038.
[37]
Górecki, J., Hofert, M., Holeňa, M. (2017),
Kendall's tau and agglomerative clustering for structure determination of hierarchical Archimedean copulas,
Dependence Modeling, 5(1), 75–87,
doi:10.1515/demo-2017-0005.
[36]
Górecki, J., Hofert, M., Holeňa, M. (2017),
On structure, family and parameter estimation of hierarchical Archimedean copulas,
Journal of Statistical Computation and Simulation, 87(17), 3261–3324,
doi:10.1080/00949655.2017.1365148.
[35]
Hofert, M., Schepsmeier, U. (2017),
International Chinese Statistical Association Bulletin,
Guidelines for statistical projects: Coding and Typography (Part III), 29(2), 113–122,
link.
[34]
Hofert, M., Schepsmeier, U. (2017),
International Chinese Statistical Association Bulletin,
Guidelines for statistical projects: Coding and Typography (Part II), 29(1), 52–58,
link.
[33]
Hofert, M., Memartoluie, A., Saunders, D., Wirjanto, T. (2017),
Improved Algorithms for Computing Worst Value-at-Risk,
Statistics & Risk Modeling, 34(1-2), 13–31,
doi:10.1515/strm-2015-0028.
[32]
Cambou, M., Lemieux, C., Hofert,
M. (2016),
Quasi-random numbers for copula models,
Statistics
and Computing, 27(5), 1307–1329,
doi:10.1007/s11222-016-9688-4.
[31]
Hofert, M., Hornik, K. (2016), How we R on
Android, Linux Journal, 6(266), 90–121.
[30]
Hofert, M., Mächler, M. (2016),
Parallel and
other simulations in R made easy: An end-to-end study,
Journal
of Statistical Software, 69(4),
doi:10.18637/jss.v069.i04.
[29]
Embrechts, P., Hofert, M., Wang. R. (2016),
Bernoulli and Tail-Dependence
Compatibility,
The Annals of Applied Probability, 26(3),
1636–1658,
doi:10.1214/15-AAP1128.
[28]
Hofert, M., Schepsmeier, U. (2016),
International Chinese Statistical Association Bulletin,
Guidelines for statistical projects: General Aspects (Part I), 28(2), 110–116,
link.
[27]
Chavez-Demoulin, V., Embrechts, P., Hofert, M. (2015),
An extreme value approach for modeling operational risk
losses depending on covariates,
Journal of Risk and Insurance
83(3), 735–776,
doi:10.1111/jori.12059.
[26]
Górecki, J., Hofert, M., Holeňa, M. (2015),
An Approach to Structure Determination and
Estimation of Hierarchical Archimedean Copulas and Its Application in Bayesian
Classification,
Journal of Intelligent Information Systems, 1–39,
doi:10.1007/s10844-014-0350-3.
[25]
[24]
Grothe, O., Hofert, M. (2014),
Construction and
sampling of Archimedean and nested Archimedean Lévy copulas,
Journal of Multivariate
Analysis,
doi:10.1016/j.jmva.2014.12.004.
[23]
Hofert, M., Mächler, M. (2013),
A
graphical goodness-of-fit test for dependence models in higher
dimensions,
Journal of Computational and Graphical
Statistics, 23(3), 700–716,
doi:10.1080/10618600.2013.812518.
[22]
Hofert, M., McNeil, A. J. (2015),
Subadditivity of
Value-at-Risk for Bernoulli random variables,
Statistics &
Probability Letters, 98, 79–88,
doi:10.1016/j.spl.2014.12.016.
[21]
Embrechts, P., Hofert, M. (2013),
A note on
generalized inverses,
Mathematical Methods of Operations
Research, 77(3), 423–432,
doi:10.1007/s00186-013-0436-7.
[20]
Embrechts, P., Hofert, M. (2013),
Statistical
inference for copulas in high dimensions: A simulation
study,
ASTIN Bulletin, 43(2), 81–95,
doi:10.1017/asb.2013.6.
[19]
Hofert, M. (2013),
On Sampling from the
Multivariate t Distribution,
The R Journal, 5(2),
129–136,
PDF.
[18]
[17]
[16]
Hofert, M., Mächler, M., McNeil,
A. J. (2013),
Archimedean Copulas in High Dimensions: Estimators and
Numerical Challenges Motivated by Financial Applications,
Journal de la Société Française de
Statistique, 154(1), 25–63,
PDF.
[15]
Hofert, M. (2012),
A stochastic representation and
sampling algorithm for nested Archimedean copulas,
Journal of
Statistical Computation and Simulation, 82(9), 1239–1255,
doi:10.1080/00949655.2011.574632.
[14]
Hofert, M. (2012),
Sampling exponentially tilted
stable distributions,
ACM Transactions on Modeling and Computer
Simulation, 22(1),
doi:10.1080/00949655.2011.574632.
[13]
Hofert, M., Wüthrich, M. V. (2012),
Statistical
Review of Nuclear Power Accidents,
Asia-Pacific Journal of Risk
and Insurance, 7(1),
doi:10.1515/2153-3792.1157.
[12]
Hofert, M., Mächler, M., McNeil,
A. J. (2012),
Likelihood inference for Archimedean copulas in high
dimensions under known margins,
Journal of Multivariate
Analysis, 110, 133–150,
doi:10.1016/j.jmva.2012.02.019.
[11]
Embrechts, P.,Hofert, M. (2011),
Comments on:
Inference in multivariate Archimedean
copula models,
TEST, 20(2), 263–270,
doi:10.1007/s11749-011-0252-4.
[10]
Embrechts, P., Hofert, M. (2011),
Practices and issues in operational risk modeling under Basel II,
Lithuanian Mathematical Journal, 51(2), 180–193,
doi:10.1007/s10986-011-9118-4.
[09]
Hofert, M. (2011),
Efficiently sampling nested Archimedean copulas,
Computational Statistics & Data Analysis, 55, 57–70,
doi:10.1016/j.csda.2010.04.025.
[08]
Hofert, M., Mächler, M. (2011),
Nested Archimedean Copulas Meet R: The nacopula Package,
Journal of Statistical Software, 39(9), 1–20,
https://www.jstatsoft.org/v39/i09/.
[07]
Hofert, M., Scherer, M. (2011),
CDO pricing with nested Archimedean copulas,
Quantitative Finance, 11(5), 775–787,
doi:10.1080/14697680903508479.
[06]
Durante, F., Hofert, M., Scherer, M. (2010),
Multivariate Hierarchical Copulas with Shocks,
Methodology and Computing in Applied Probability, 12(4), 681–694,
doi:10.1007/s11009-009-9134-6.
[05]
Hering, C., Hofert, M., Mai, J.-F., Scherer, M. (2010),
Constructing nested Archimedean copulas with Lévy subordinators,
Journal of Multivariate Analysis, 101, 1428–1433,
doi:10.1016/j.jmva.2009.10.005.
[04]
[03]
Hofert, M., Kohm, M. (2010),
Scientific Presentations with LATEX,
The PracTEX Journal, 2,
link.
[02]
Hofert, M., Scherer, M., Zagst, R. (2010),
Modeling the evolution of implied CDO correlations,
Financial Markets and Portfolio Management, 24(3), 289–308,
doi:10.1007/s11408-010-0136-8.
[01]
Book Contributions
[07]
Embrechts, P., Hofert, M. (2012), Risk Measures and Dependence Modeling, Handbook of Insurance, edition 3, ed. by Dionne, G., Springer, to appear in 2024.
[06]
Hintz, E., Hofert, M., Lemieux, C. (2022), Quasi-random sampling with black box or acceptance-rejection inputs, Advances in Modeling and Simulation, ed. by Botev, Z., Keller, A., Lemieux, C., Tuffin, B., Springer, 261–281.
[05]
Arbenz, P., Cambou, M., Hofert, M., Lemieux, C., Taniguchi, Y. (2018), Importance Sampling and Stratification for Copula Models, Contemporary Computational Mathematics – a celebration of the 80th birthday of Ian Sloan, ed. by Dick, J., Kuo, F. Y., Woźniakowski, H.
[04]
Górecki, J., Hofert, M., Holeňa, M. (2014),
On the consistency of an estimator for hierarchical Archimedean copulas,
32nd International Conference on Mathematical Methods in Economics, ed. by Talašová, J., Stoklasa, J., Talášek, T., Palacký University, Olomouc, 239–244,
link.
[03]
Hering, C., Hofert, M. (2012), Goodness-of-fit tests for Archimedean copulas in high dimensions, Innovations in Quantitative Risk Management, ed. by Glau, K., Scherer, M., Zagst, R., Springer, 357–373.
[02]
Embrechts, P., Hofert, M. (2012), Risk Measures and Dependence Modeling, Handbook of Insurance, edition 2, ed. by Dionne, G., Springer, 135–166.
[01]
Hofert, M. (2010),
Construction and sampling of nested
Archimedean copulas,
Copula Theory and Its Applications,
Proceedings of the Workshop held in Warsaw 25–26 September 2009,
ed. by Durante, F., Härdle, W., Jaworski, P., Rychlik, T.,
Springer,
147–160,
doi:10.1007/978-3-642-12465-5_7.
Books
[04]
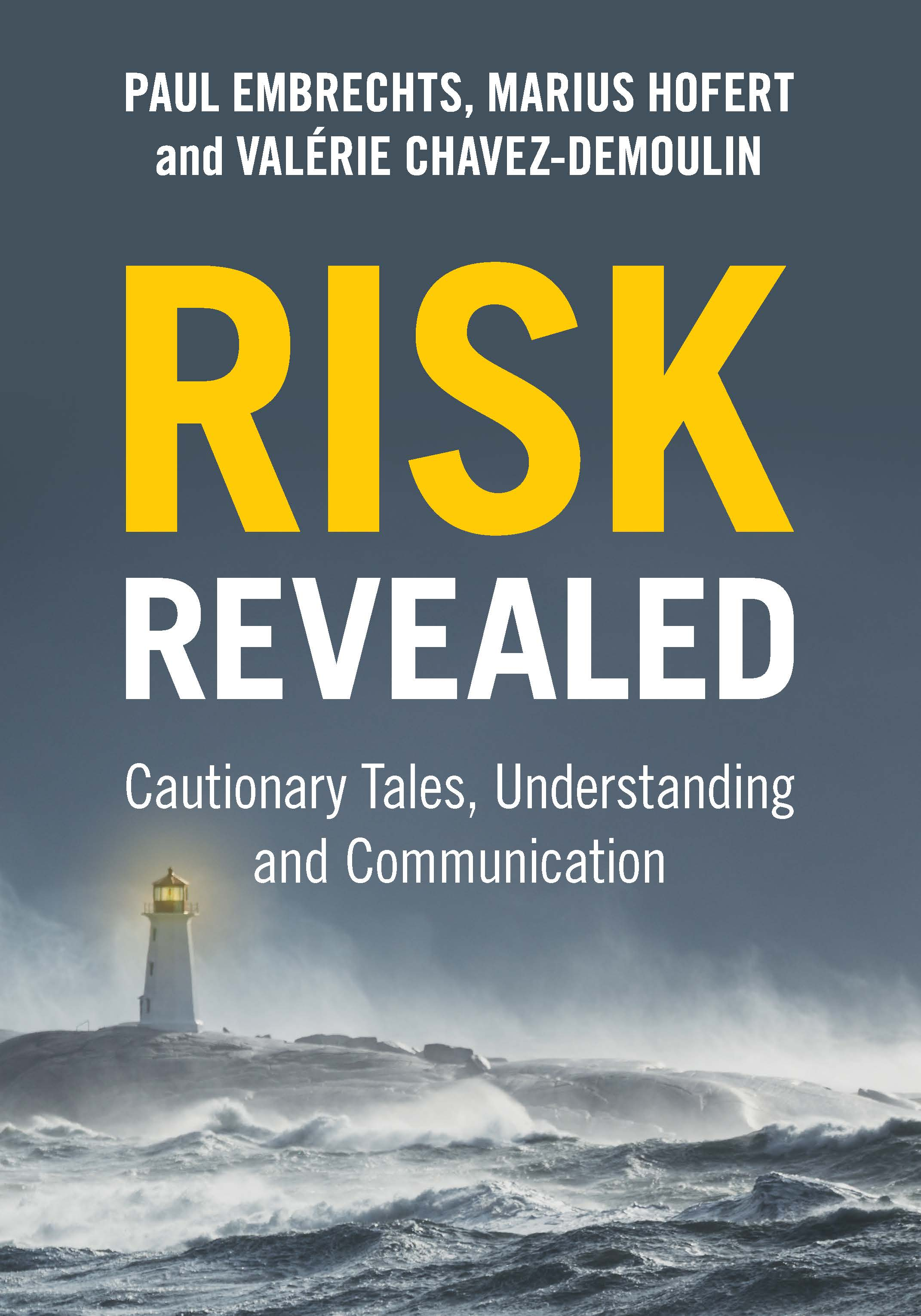
Embrechts, P., Hofert, M., Chavez-Demoulin, V. (2023),
Risk Revealed: Cautionary Tales, Understanding and Communication,
Cambridge University Press, to appear in mid 2024.
[03]
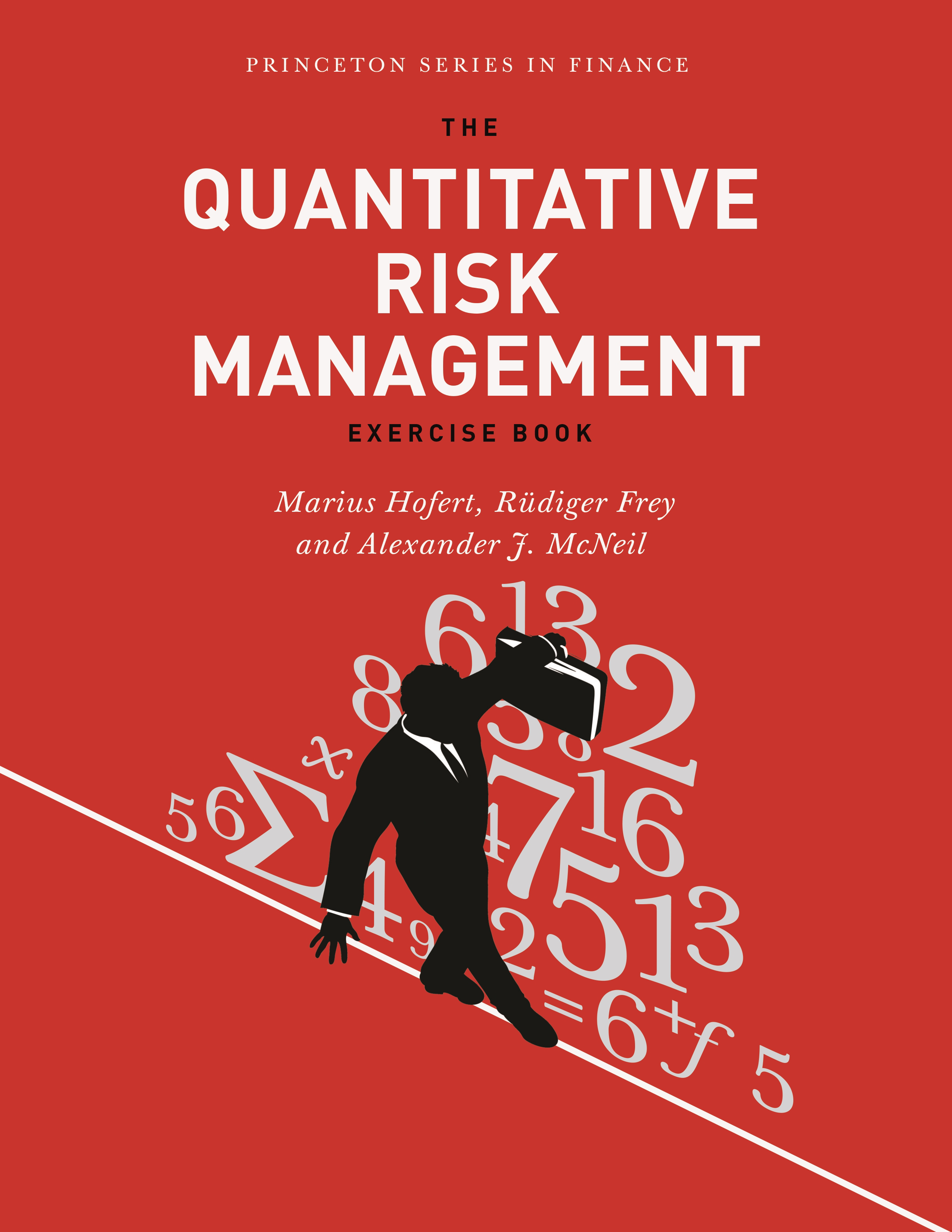
Hofert, M., Frey, R., McNeil, A. J. (2020),
The Quantitative Risk Management Exercise Book,
Princeton University Press,
ISBN 9780691206707.
For the solution manual, see
here
and for the solution to R exercises, see
here.
Cite the book via:
@Book{,
publisher = {Princeton University Press},
year = 2020,
isbn = {9780691206707},
url = {https://assets.press.princeton.edu/releases/2019-12-03_The_QRM_Exercise_Book.pdf},
title = {{T}he {Q}uantitative {R}isk {M}anagement {E}xercise {B}ook},
author = {Hofert, M., Frey, R., McNeil, A. J.},
}
[02]
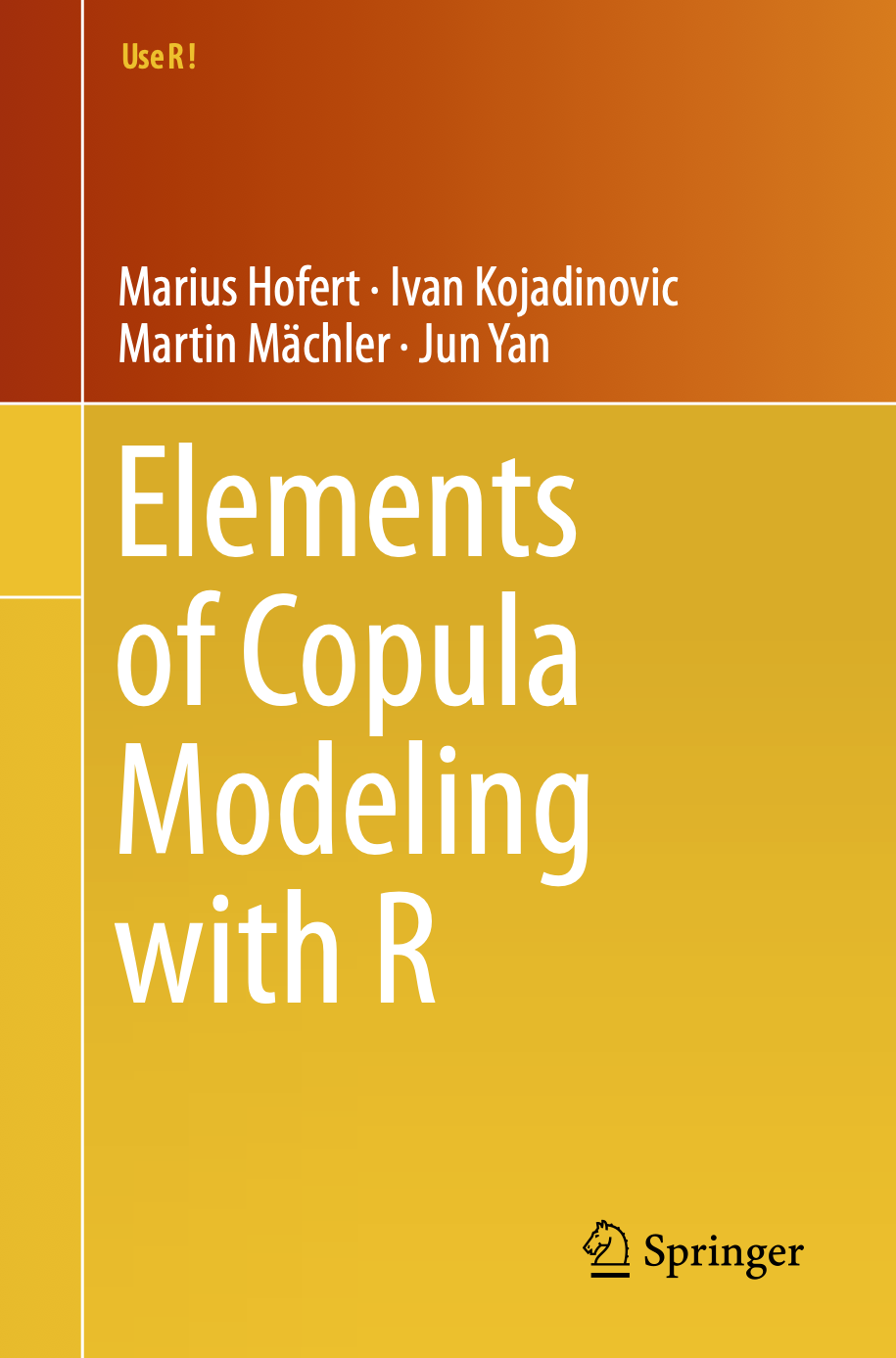
Hofert, M., Kojadinovic, I., Mächler, M., Yan, J. (2018),
Elements of Copula Modeling with R,
Springer Use R! Series,
ISBN 978-3-319-89635-9.
For the code and more, see
here.
For the Use R! Springer book page, see
here.
Cite the book via:
@Book{,
publisher = {Springer Use R! Series},
year = 2018,
isbn = {978-3-319-89635-9},
url = {https://www.springer.com/de/book/9783319896342},
title = {{E}lements of {C}opula {M}odeling with \textsf{R}},
author = {Hofert, M., Kojadinovic, I., Maechler, M., Yan, J.},
}
[01]
Hofert, M. (2010),
Sampling Nested Archimedean Copulas with Applications to CDO Pricing, PhD thesis,
Südwestdeutscher Verlag für Hochschulschriften AG & Co. KG,
ISBN 978-3-8381-1656-3.
Miscellaneous
[01]
Hofert, M., Schepsmeier, U.
Guidelines
for Statistical Projects: Coding and Typography,
PDF.
- Rüdiger Frey, Alexander J. McNeil and I maintain
a website about research and teaching material on
Quantitative Risk Management (QRM): http://www.qrmtutorial.org.
Software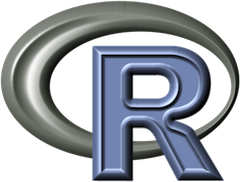
The software (including book-length manuals, examples, vignettes,
demos, tests, etc.) is provided for free. Feel free to use it and cite
the software properly (see below). Note that we are not required to
provide support (such as solving concrete assignment problems, writing
Master's or PhD theses or providing modeling support and engines for
companies). The software is open source, so you can also study the
source code to solve your problem. Alternatively, ask on major mailing lists (e.g.,
R help) or software-related forums
(e.g., Stack Overflow). If you are convinced you found a bug,
write to maintainer() and provide
a minimal working example.
-
R package copula
The first R package on copulas. It covers a wide
range of statistical tasks in copula modeling.
Current stable: https://cran.r-project.org/package=copula
Latest development: https://r-forge.r-project.org/projects/copula
Cite the package via:
@Manual{,
title = {copula: Multivariate Dependence with Copulas},
author = {Hofert, M., Kojadinovic, I., M{\"a}chler, M., Yan, J.},
year = {2016},
doi = {10.32614/CRAN.package.copula},
note = {R package version 0.999-20},
url = {https://cran.r-project.org/package=copula}
}
-
R package copulaData
Datasets for copula modeling.
Current stable: https://cran.r-project.org/package=copulaData
Latest development: https://r-forge.r-project.org/projects/copula
Cite the package via:
@Manual{,
title = {copulaData: Data Sets for Copula Modeling},
author = {Hofert, M., Kojadinovic, I., M{\"a}chler, M., Yan, J.},
year = {2018},
doi = {10.32614/CRAN.package.copulaData},
note = {R package version 0.0-1},
url = {https://cran.r-project.org/package=copulaData}
}
-
R package crop
An R package for exactly cropping graphics (.png, .pdf, .ps, .eps) from within
R via underlying Unix tools.
Current stable: https://cran.r-project.org/package=crop
Latest development: https://r-forge.r-project.org/projects/crop/
Cite the package via:
@Manual{,
title = {crop: Graphics Cropping Tool},
author = {Hofert, M.},
year = {2015},
doi = {10.32614/CRAN.package.crop},
note = {R package version 0.0-2},
url = {https://cran.r-project.org/package=crop}
}
-
R package gnn
An R package for generative neural network modeling.
Current stable: https://cran.r-project.org/package=gnn
Latest development: https://r-forge.r-project.org/projects/gnn
Cite the package via:
@Manual{,
title = {gnn: Generative Neural Networks},
author = {Hofert, M., Prasad, A.},
year = {2019},
doi = {10.32614/CRAN.package.gnn},
note = {R package version 0.0-2},a
url = {https://cran.r-project.org/package=gnn}
}
-
R package nvmix
Multivariate normal variance mixtures (including Student t distribution for non-integer degrees of freedom).
Current stable: https://cran.r-project.org/package=nvmix
Latest development: https://r-forge.r-project.org/projects/nvmix
Cite the package via:
@Manual{,
title = {nvmix: Multivariate Normal Variance Mixtures (Including Student's t Distribution for Non-Integer Degrees of Freedom)},
author = {Hofert, M., Hintz, E., Lemieux, C.},
year = {2018},
doi = {10.32614/CRAN.package.nvmix},
note = {R package version 0.0-3},
url = {https://cran.r-project.org/package=nvmix}
}
-
R package qrmdata
An R package of data sets useful in Quantitative Risk Management.
Current stable: https://cran.r-project.org/package=qrmdata
Latest development: https://r-forge.r-project.org/projects/qrmdata
Cite the package via:
@Manual{,
title = {qrmdata: Data Sets for Quantitative Risk Management Practice},
author = {Hofert, M., Hornik, K.},
year = {2016},
doi = {10.32614/CRAN.package.qrmdata},
note = {R package version 2019-12-03-1},
url = {https://cran.r-project.org/package=qrmdata}
}
-
R package qrmtools
An R package providing selected unctionality around QRM.
Current stable: https://cran.r-project.org/package=qrmtools
Latest development: https://r-forge.r-project.org/projects/qrmtools
Cite the package via:
@Manual{,
title = {qrmtools: Tools for Quantitative Risk Management},
author = {Hofert, M., Hornik, K.},
year = {2016},
doi = {10.32614/CRAN.package.qrmtools},
note = {R package version 0.0-12},
url = {https://cran.r-project.org/package=qrmtools}
}
-
R package qrng
An R package providing selected quasi-random number generators.
Current stable: https://cran.r-project.org/package=qrng
Latest development: https://r-forge.r-project.org/projects/qrng
Cite the package via:
@Manual{,
title = {qrng: (Randomized) Quasi-Random Number Generators},
author = {Hofert, M., Lemieux, C.},
year = {2016},
doi = {10.32614/CRAN.package.qrng},
note = {R package version 0.0-7},
url = {https://cran.r-project.org/package=qrng}
}
-
R package simsalapar
An R package for conducting large-scale simulation
studies in R (including error/warning catching, run time
measurement, parallel computing, and more).
Current stable: https://cran.r-project.org/package=simsalapar
Cite the package via:
@Manual{,
title = {simsalapar: Tools for Simulation Studies in Parallel with R},
author = {Hofert, M., M{\"a}chler, M.},
year = {2016},
doi = {10.32614/CRAN.package.simsalapar},
note = {R package version 1.0-10},
url = {https://cran.r-project.org/package=simsalapar}
}
-
Packages I originally authored or contributed to:
mvtnorm,
QRM,
zenplots